Department Highlights
Three New Faculty Members to Join the Department in August
Xingche Guo (Statistical Machine Learning), Trevor Harris (cluster hire in Artificial Intelligence/Machine Learning: Understanding and Addressing Natural and Climate Change-Related Disasters), and Wenrui Li (Modern Statistical Computing) will start as assistant professors of statistics this fall.
Two New Undergraduate Degrees in Data Science
Learn more about the Department's new Bachelor of Science (BS) in Statistical Data Science.
Upcoming Local Conferences
Learn more about a range of upcoming academic conferences organized by UConn statistics department faculty
News and Announcements
Upcoming Events
-
May
9
The Stat4Onc Annual Symposium All Day
The Stat4Onc Annual Symposium
Thursday, May 9th, 2024
All Day
The Stat4Onc Annual Symposium is a venue for interdisciplinary dialogue among clinical and quantitative scientists about cancer clinical trials. We seek participation by researchers from academia, industry, and regulatory agencies to share new research, discuss novel ideas, ask questions, and provide solutions for cancer clinical trials. Oncologists and statisticians can exchange views on trial design and conduct, drug development, and translation to patient care. Topics for this symposium include training for new generation of statisticians and clinicians, translational and precision oncology, master protocols, pediatric oncology research and drug development, populational cancer research, biomarkers and subgroups, real world data/evidence, and engagement of under-representative population in oncology research.Please visit the event’s homepage for this information at https://stat4onc.org/events/stat4onc2024/. -
May
21
New England Statistics Symposium All Day
New England Statistics Symposium
Tuesday, May 21st, 2024
All Day
The symposium has been bringing together statisticians from all over New England and beyond to a central location to share research, discuss emerging issues in the field, and network with colleagues since it was first started at UConn in 1987.Please visit the event’s homepage for this information at -
May
22
New England Statistics Symposium All Day
New England Statistics Symposium
Wednesday, May 22nd, 2024
All Day
The symposium has been bringing together statisticians from all over New England and beyond to a central location to share research, discuss emerging issues in the field, and network with colleagues since it was first started at UConn in 1987.Please visit the event’s homepage for this information at
Academic Programs
The Department of Statistics provides outstanding undergraduate and graduate education to students at UConn, preparing them for successful careers in academia, industry, government, and beyond.
Quick Links
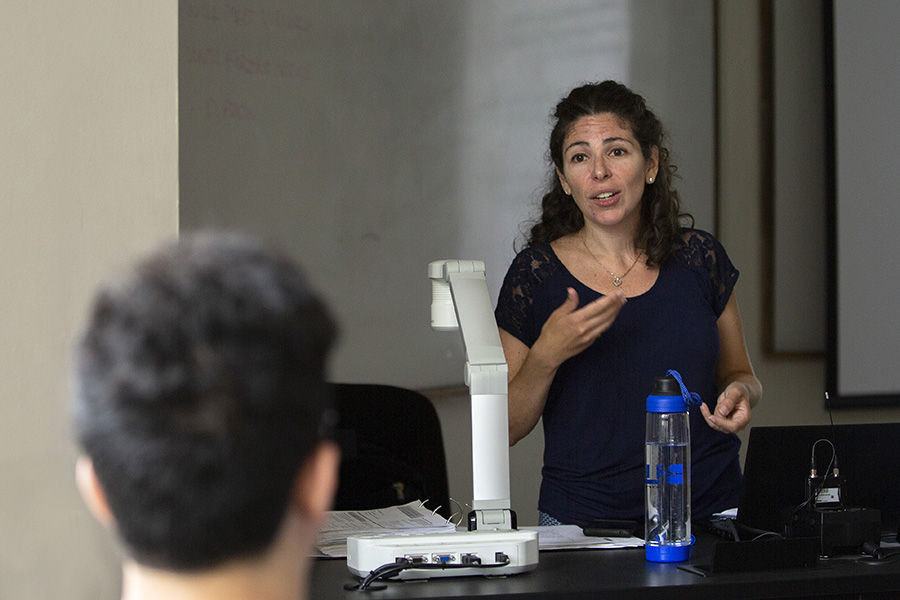
Undergraduate Advising
Our advisors help students navigate their requirements, select courses, and make the most of their academic experience.
Make an Appointment
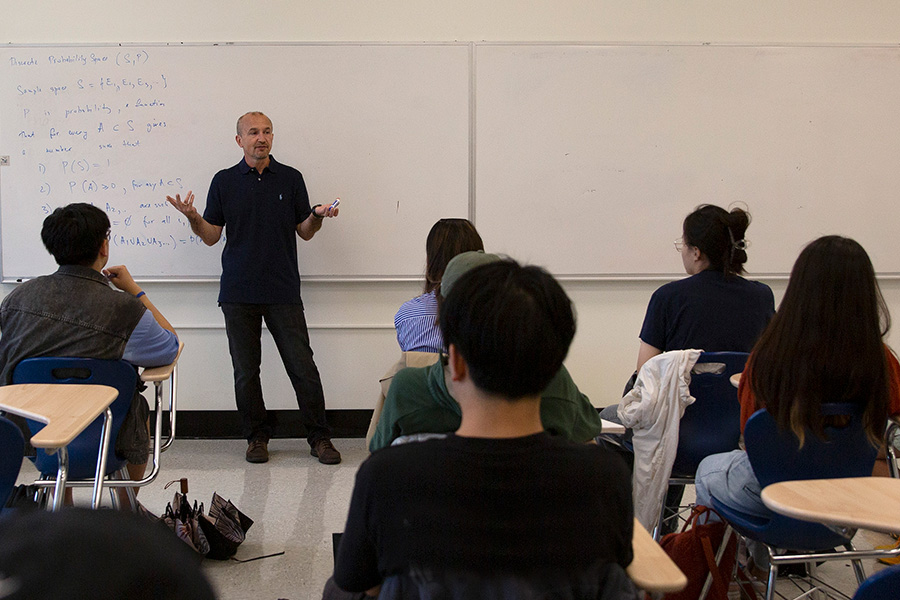
Graduate Education
The Department of Statistics welcomes applicants to our Master of Science (MS) and Doctor of Philosophy (Ph.D.) programs.
Compare Programs
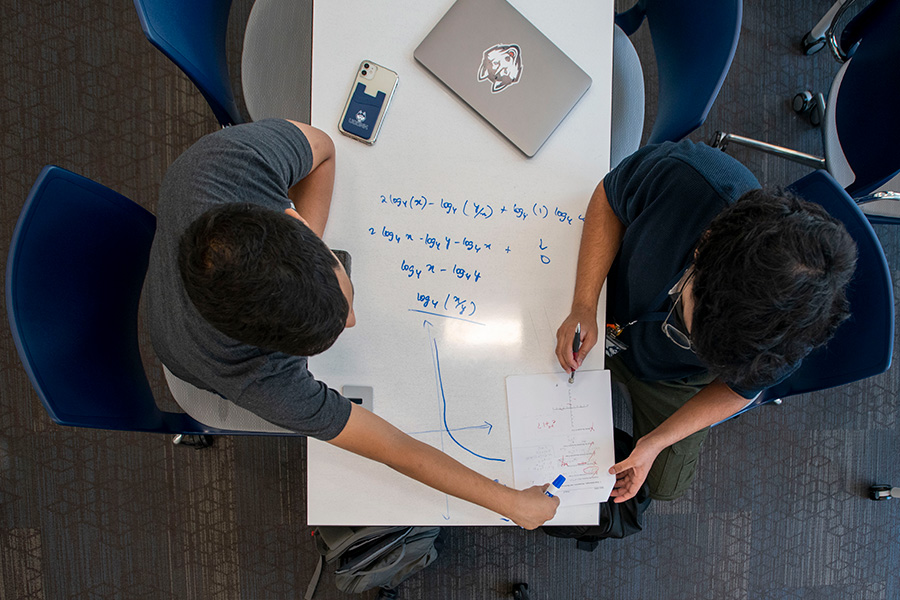
Outreach and Engagement
Through events and outreach initiatives, we share our expertise with students and scholars at UConn and beyond.
Our initiatives
Recent Events
The Department hosts events that contribute new knowledge, stimulate discussions, and build community among scholars and students across the University.
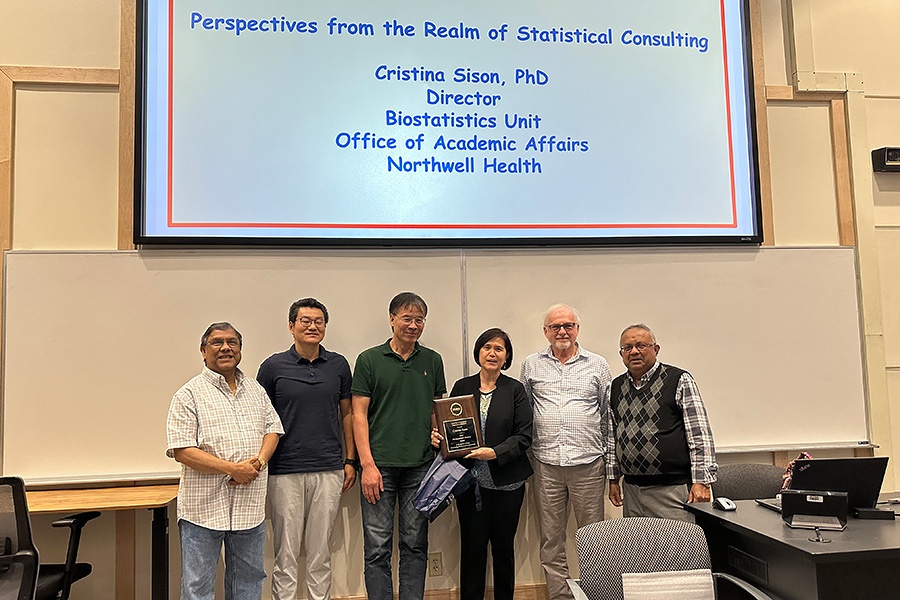
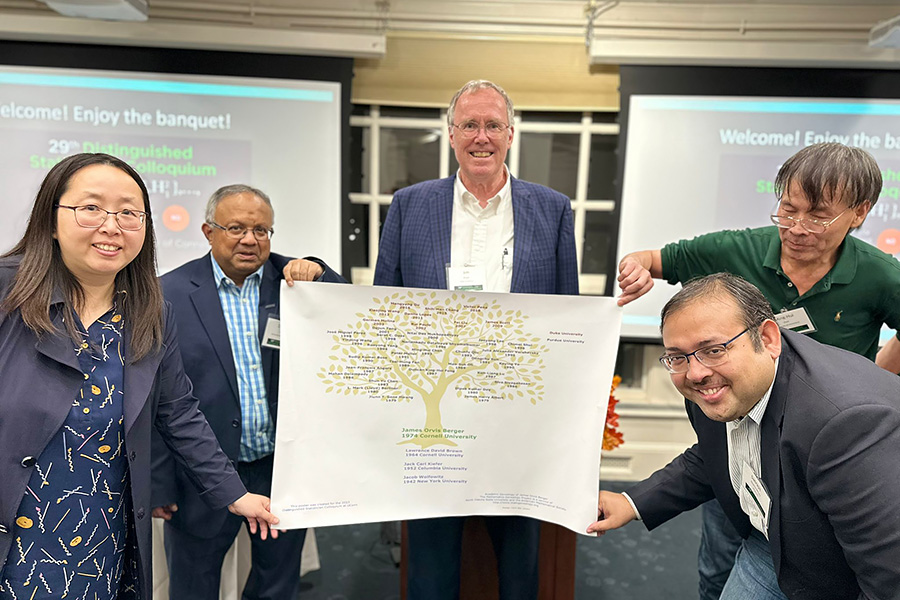
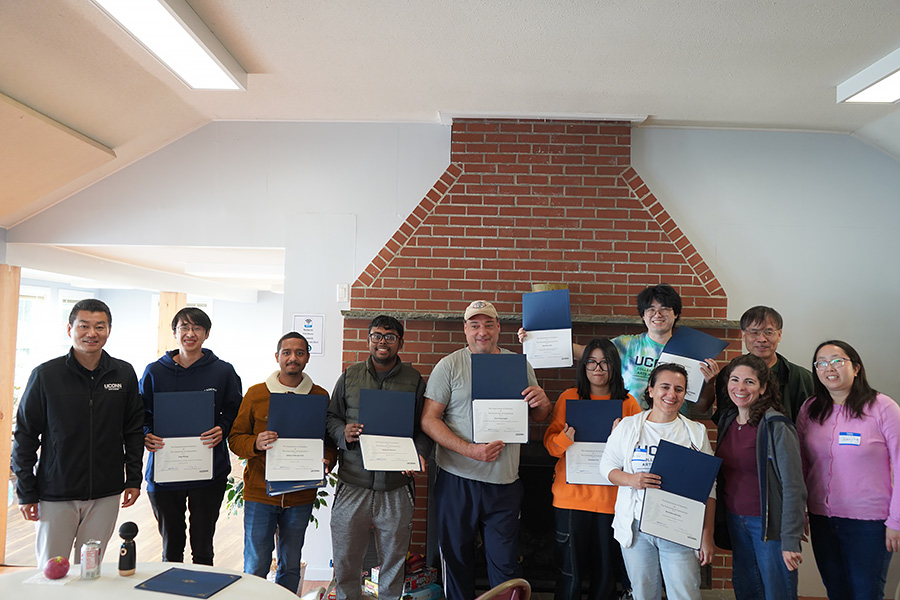